This article appears in the Fall-Winter 2019 digital issue of DOCUMENT Strategy. Subscribe.

Unfortunately, those heat maps rely on what can be called “pseudo-quantitative” methods, in which risks, benefits, and other factors are numerically rated. While the output can look scientific, the rankings are arbitrary and subjective. In fact, there is zero empirical evidence that such rankings are accurate and many fundamental reasons why they aren’t.
According to research conducted by Douglas Hubbard, author of How to Measure Anything: Finding the Value of Intangibles in Business and inventor of the Applied Information Economics method, random guessing actually produces better results. Therefore, using heat maps is less than useless; it’s harmful.
The reason why heat maps don’t work isn’t complex. Assigning ordinal numbers to qualitative ratings is about as accurate as using hotel star reviews, but with much more serious implications. Just because you have assigned a number to something doesn’t mean you can calculate a numerical solution from it. Does an event that causes a large financial loss rated as a “4” have exactly twice the impact of a medium financial loss assigned a “2” rating?
Heat maps also imply a level of certainty that rarely exists. The reality is that the possible outcomes of a risk event are uncertain and exist on a spectrum. There is no way to express all of this with a single dot on a heat map. To express risk in business terms, a cybersecurity professional needs a probabilistic method.

Image by: diki darmawan, ©2019 Getty Images
These days, there’s no shortage of news stories about the latest data breach, which may leave a lot of cybersecurity professionals in a cold sweat more frequently. Furthermore, some of these professionals and chief information officers (CIOs) are under pressure from their boards and regulators to materially assess their cyber risk—something traditionally accomplished with cyber risk heat maps.
Unfortunately, those heat maps rely on what can be called “pseudo-quantitative” methods, in which risks, benefits, and other factors are numerically rated. While the output can look scientific, the rankings are arbitrary and subjective. In fact, there is zero empirical evidence that such rankings are accurate and many fundamental reasons why they aren’t.
According to research conducted by Douglas Hubbard, author of How to Measure Anything: Finding the Value of Intangibles in Business and inventor of the Applied Information Economics method, random guessing actually produces better results. Therefore, using heat maps is less than useless; it’s harmful.
The reason why heat maps don’t work isn’t complex. Assigning ordinal numbers to qualitative ratings is about as accurate as using hotel star reviews, but with much more serious implications. Just because you have assigned a number to something doesn’t mean you can calculate a numerical solution from it. Does an event that causes a large financial loss rated as a “4” have exactly twice the impact of a medium financial loss assigned a “2” rating?
Heat maps also imply a level of certainty that rarely exists. The reality is that the possible outcomes of a risk event are uncertain and exist on a spectrum. There is no way to express all of this with a single dot on a heat map. To express risk in business terms, a cybersecurity professional needs a probabilistic method.
What’s the bottom line? Too often, heat maps merely document gut feelings and are designed to justify the decisions cybersecurity leaders have already made.
However, there is a better cyber risk quantification method that can provide an organization with greater confidence in their risk assessments. A quantitative assessment model, such as the Factor Analysis of Information Risk (FAIR), can be used to express the financial loss exposure of a cyber threat scenario through probabilistic analysis, providing a standard risk language to ensure consistency. Likewise, an analyst using FAIR can demonstrate how established controls reduce risk, thereby, evaluating potential investments in cybersecurity technology at the same time.
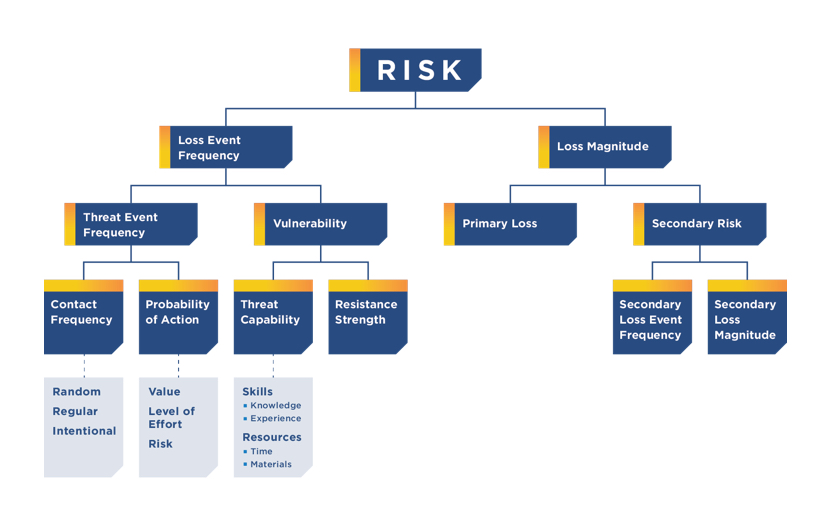
To use these kinds of models, like FAIR, the first step is to clearly delineate a variety of threat events, from weather to malicious actors, and the assets of business value that could be threatened. The cybersecurity function will need to gather the data required to populate the FAIR model by holding workshops with subject matter experts, examining existing and proposed policies and standards and reviewing system-generated reports, management reports, and manually collected metrics.
Using the Monte Carlo simulation, an analyst can then run thousands of simulations for the threat event, creating a distribution of probable losses. For organizations new to quantitative modeling, this process can be challenging because the scope or scenario often lack clear and proper definitions. These speed bumps are easily overcome with guidance and experience.
Unlike heat maps, Monte Carlo simulations do math with uncertain inputs and, in turn, show the uncertainty in the results. This quantification of risk gives decision makers a much clearer view of threats they are facing, the financial stakes at risk, and the degree of confidence that should be assigned to the outcomes. It also reveals the relative benefits of mitigating those threats.
While adopting FAIR or another quantitative model may appear daunting to organizations that have utilized heat maps for years, it’s hard to justify not transitioning to these approaches when the benefits are considered. In addition to understanding the cost of different events and clarifying the return on investment to mitigate them, quantitative models provide complete cybersecurity assessments at a lower cost with better results than heat maps. They also help organizations prioritize security concerns in monetary terms, determine the appropriate amount of insurance, make better decisions, and comply with regulations.
However, there is a better cyber risk quantification method that can provide an organization with greater confidence in their risk assessments. A quantitative assessment model, such as the Factor Analysis of Information Risk (FAIR), can be used to express the financial loss exposure of a cyber threat scenario through probabilistic analysis, providing a standard risk language to ensure consistency. Likewise, an analyst using FAIR can demonstrate how established controls reduce risk, thereby, evaluating potential investments in cybersecurity technology at the same time.
The FAIR Model
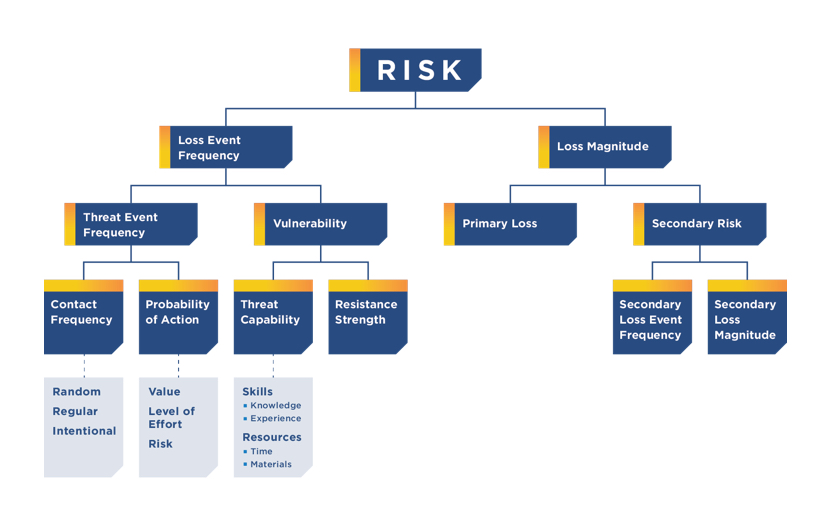
The Factor Analysis of Information Risk (FAIR) is a quantitative model for information security and operational risk.
For more information, please visit www.fairinstitute.org.
To use these kinds of models, like FAIR, the first step is to clearly delineate a variety of threat events, from weather to malicious actors, and the assets of business value that could be threatened. The cybersecurity function will need to gather the data required to populate the FAIR model by holding workshops with subject matter experts, examining existing and proposed policies and standards and reviewing system-generated reports, management reports, and manually collected metrics.
Using the Monte Carlo simulation, an analyst can then run thousands of simulations for the threat event, creating a distribution of probable losses. For organizations new to quantitative modeling, this process can be challenging because the scope or scenario often lack clear and proper definitions. These speed bumps are easily overcome with guidance and experience.
Unlike heat maps, Monte Carlo simulations do math with uncertain inputs and, in turn, show the uncertainty in the results. This quantification of risk gives decision makers a much clearer view of threats they are facing, the financial stakes at risk, and the degree of confidence that should be assigned to the outcomes. It also reveals the relative benefits of mitigating those threats.
While adopting FAIR or another quantitative model may appear daunting to organizations that have utilized heat maps for years, it’s hard to justify not transitioning to these approaches when the benefits are considered. In addition to understanding the cost of different events and clarifying the return on investment to mitigate them, quantitative models provide complete cybersecurity assessments at a lower cost with better results than heat maps. They also help organizations prioritize security concerns in monetary terms, determine the appropriate amount of insurance, make better decisions, and comply with regulations.
Vince Dasta is the Associate Director of the Security and Privacy practice at Protiviti, where he helps companies quantify and manage their cybersecurity risk. For more information, visit www.protiviti.com.