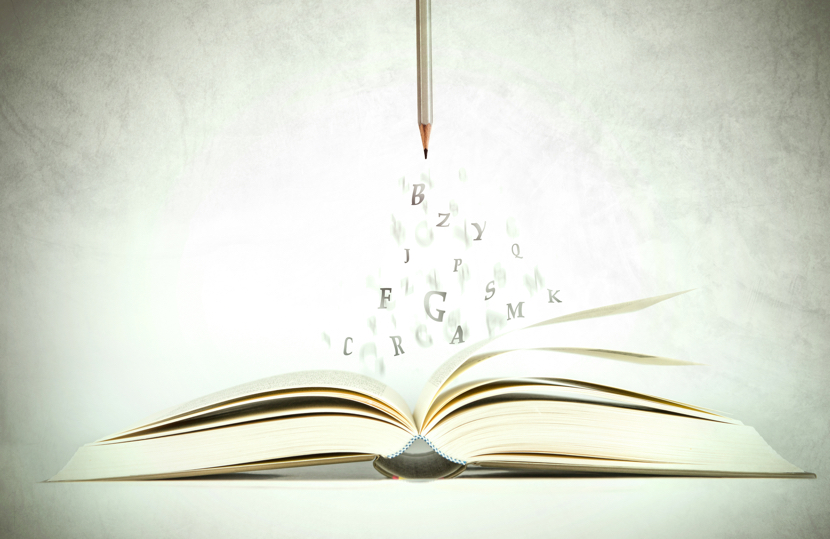
Image by: pinkomelet, ©2017 Getty Images
Walk through any expo floor and chances are that you will see a half a dozen vendors touting “content analytics." In document management, it's the cool “new” topic. The problem is that content analytics is not new, and often, many of the vendors promoting its support use it themselves. Content analytics has been around for almost 18 years. It can change the way organizations work not only with their documents but the information stored in those documents.
It is often easier to explain what content analytics is not than what it is.
Content Analytics Is Not Zonal OCR or Full-Text Search
One of the biggest challenges in understanding content analytics is that too many technologies want to say that they perform content analytics. So, let’s start with a definition: Content analytics uses tools and techniques to extract meaning out of documents or other content sources. Using a very loose definition, things like zonal optical character recognition (OCR) or full-text search would fall into this category, but these weren't called “content analytics” two years ago.With zonal OCR, individuals define a location on a page to be scanned to search for specific information based on recognized patterns. Full-text search gets a little closer. Concept searching performs pattern recognition, but it often includes vocabularies to recognize concepts like tenses. However, these technologies have been mainstream in document and content management for several decades. The attempt to make them fresh by capitalizing on this term hinders the adoption of real content analytics.
Content Analytics Is Based on Computational Linguistics
The current generation of content analytics uses concepts of computational linguistics to look at content. Computational linguistics is a field of its own, which uses computer science principles to analyze written and spoken language. It looks at grammar tense, or morphology, and sentence syntax. It also considers computation semantics—the fact that a single word may have multiple meanings. For instance, a “bank” can mean a financial institution, the edge of a river, the turning of an aircraft, or using the rail in a game of billiards.The most common use of computational linguistics has been in the field of machine translation—also called automated linguistic translation. Machine translation looks at the entire sentence to translate one language into another. Computational linguistics is used to process the sentence or phrase into the different parts of speech and then return a translated copy (e.g., US English to Canadian French).
In the past, content analytics has been used to group like documents for categorization or classification (affinity groups) or to summarize large documents (document essence). The latest examples is found around identifying document outliers or fraud detection. When identifying a document outlier, content analytics attempts to match a document to its expected classification. If a document falls too far outside this classification, it is flagged as an outlier. The key to mapping the document relationship comes from Word2Vec.
Content Analytics Is About Word2Vec
The primary concept used to perform such processes is Word2Vec (think words to vector). It looks at each individual word and performs an analysis to plot it in a three-dimensional space, or vector, based on a computed algorithm. The computations are such that when these words are plotted in a three-dimensional space, similar words are mapped in close proximity to each other. Because of this, even jargon can be plotted using Word2Vec. These vector maps are then used to identify like terms as well as outliers. Word2Vec concepts have been extended to Doc2Vec, which looks at documents in the same way.Putting Content Analytics to Use
The challenge with content analytics is that people are looking for existing use cases. Classification and categorization are simple examples that exist today. For content analytics to really take off, it needs ideas for implementation. Information technology (IT) can tell if documents are similar, find like documents, and find documents with irregularities. These same calculations can be used on the words inside of documents, which is where content analytics shows its power. We need to understand its potential, and only then can we understand the questions that content analytics can help to answer.A showcase solution in document management was how it helped to bring Pfizer’s Viagra to the market three months earlier by improving the FDA submission process. Those three extra months meant millions of dollars in revenue, but Viagra was accidentally discovered from reactions that were reported in clinical trial documents. A showcase solution for content analytics could be finding the next new drug by reviewing collections of millions of clinical trial documents.
Marko Sillanpaa is co-founder of the blog Big Men On Content and the founder of BMO Consulting. He has been working in ECM for over 18 years for vendors like Documentum, EMC, Hyland, and SDL Trados and systems integrators like CSC and Accenture. Follow him on Twitter @MSillanpaaBMOC.