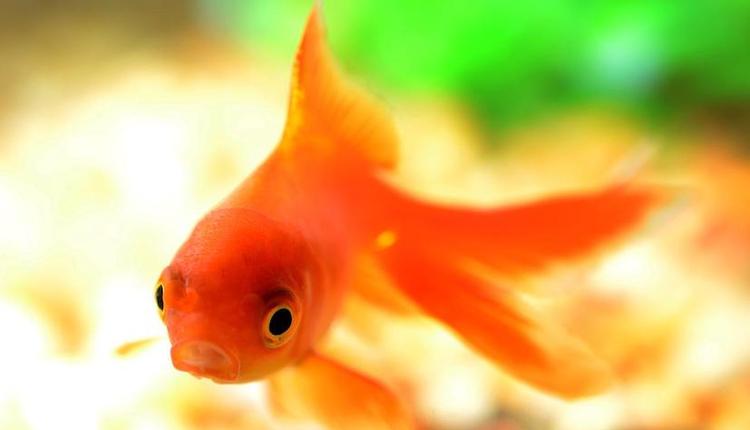
There’s a whole plethora of unicorn companies (mega-valuation start-ups) out there, whose AI-based propositions are based on pattern recognition. Far from being unintelligent robotic process automation tools, their tools train and use AI to recognize and make sense of documents and their contents. Using image/text recognition they can deduce when incoming PDFs or paper documents are invoices, for instance, and make an experienced guess (algorithm/score-based) on which of the various content parts constitute the company name, tax ID, payable value and so on. And they can go on to part-process that information in a semi-structured way (populate a database, check a box, trigger the next step in a workflow), easing the strain on busy teams and so on.
BUT, pattern matching — while great — is only half of the story. Real transformation comes only when this can be integrated with the bigger enterprise picture, enabling reliable onward decision-making and streamlining extended processes. Without that integration and wider contextualization, even the slickest intelligent order or invoice automation solution (or whatever the immediate use case) will be restricted in its potential.
Each time the system encounters a new document it will be as a goldfish, beginning its memory-less discovery again from scratch.
Without enterprise context: single-use discovery, no actionable insights
The problem in these early scenarios is that, however “smart” the AI-enabled automation tool may be, it isn’t building up exploitable, re-usable knowledge about Bates Inc/John Bates and his track record as a supplier — or conversely his buying habits as a customer (nor his payment history, nor his satisfaction rating). So it isn’t contributing to the nirvana that is the 360-degree customer/supplier view. And, unless the facility feeds straight into ERP and finance systems, it isn’t going to result in John’s latest order/payment being approved any faster, as he hasn’t been identified as a known account — and indeed his transaction can’t even be identified as non-fraudulent.
The problem is that the intelligent AI capability isn’t joining the dots. It isn’t matching orders to historic customer records or invoices to POs. The gains to the business, then, and in the form of an improved experience for John or for Bates Inc, aren’t as “transformative” as they might be.
This is the vital next step for AI in the enterprise, then: the blending of AI and latest best practice in advanced document management with — and so it contributes to — synthesized institutional knowledge. Here — across an entire platform, and multiple applications — the company’s AI-enhanced knowledge bank is enriched by every document that ever passes across it. Each one is remembered, each one adds to enterprise learning — to the bigger picture.
Turning patterns into strategic interventions
With this powerful interlay of pattern recognition and contextual AI, the scope for business process transformation increases several-fold. Now, once an incoming document has been identified, the AI-enabled content services platform knows not just what it is and what its constituent information means, but also what to do with it next — which systems need to be updated, and which next-level actions can be triggered confidently.
If it’s an invoice from a recognized supplier, and the amount tallies with the PO/with what was expected, it could be that protracted processes (sequential approvals) are now circumvented, for instance, resulting in faster payment for a loyal supplier.
As orders rack up for a recently added customer; meanwhile, discounts and other incentives could be invoked to reward loyalty — or to compensate for a recent poor experience. If it’s a HR/workforce-based scenario, this could be about building a picture from sickness days, holiday bookings, record access or meeting requests of employees who may be feeling restless, anxious or dissatisfied, allowing line managers to step in with a timely review, revised training plan, pay raise or promotion to prevent valued talent from leaving.
Where “360-degree visibility” applications fall down
Although the plethora of 360-degree CRM/SCM/ERP/HCM applications have long promised to provide such holistic intelligence, the effectiveness of these systems relies on the information that teams proactively feed them. Simply using these systems doesn’t guarantee that a sales team will know when they are wasting their time (because that customer is a lousy payer), or that there’s a related contract currently with the legal team, or that staff from that company have outstanding requests with the service desk. Add advanced, AI-enabled document intake/content management into the mix — a capability that transcends a single application or department — and that whole-picture view will be continuously enriched automatically, driving ever better decisions and more timely interventions.
Future-proofing: beware single-purpose AI
There’s another important reason why AI’s potential should be decoupled from single-purpose applications, and that’s in the interests of future-proofing. A software application that is marketed “with AI in it” is, by definition, already out of date. The technology is developing so rapidly that any capabilities embedded today will have a very limited shelf life.
Where a software application comes with “AI built in” also suggests that these software companies have bet on a particular AI/machine learning framework. That could be Google TensorFlow, Microsoft Azure Cognitive Services, a Python-based framework, or specific capabilities for pattern matching/image recognition or natural language processing (BERT, ERNIE, etc). Given how quickly things change, and leading technologies are disrupted, going all in with a single AI framework is a risky move.
Rather than standardize on any one set of capabilities, companies would probably do better to plump for an “open” content architecture, which supports any combination of current and future AI options on a “composable” basis, as needs change and as technology continues to evolve.
Time to harvest the higher fruit
Above all, as enterprises move beyond the low-hanging fruit in their AI ambitions — in other words, beyond superficial, RPA-based automation (with its emphasis on screen-scraping/cutting-and-pasting of content rather than on understanding and modeling its semantics), the focus does need to shift to cross-enterprise integration and contextualization. That’s if businesses are to exploit AI and machine learning to its fuller potential and deliver that elusive “single view” of customers/suppliers/employees/products — or whatever the strategic goal happens to be.
All kinds of developments — in the global economy, in the reinvention of the workplace, and in the world of technology — are creating the perfect storm for AI’s extended and more deeply integrated role in an organization and its processes — augmenting and accelerating the vital everyday work that human teams are working so hard to stay on top of.
It’s against that backdrop that composable, embedded, contextual AI use will drive next, more ambitious waves of intelligent content and related process automation — and the possibilities are endless.