This article appears in the Fall 2017 digital issue of DOCUMENT Strategy. Subscribe.
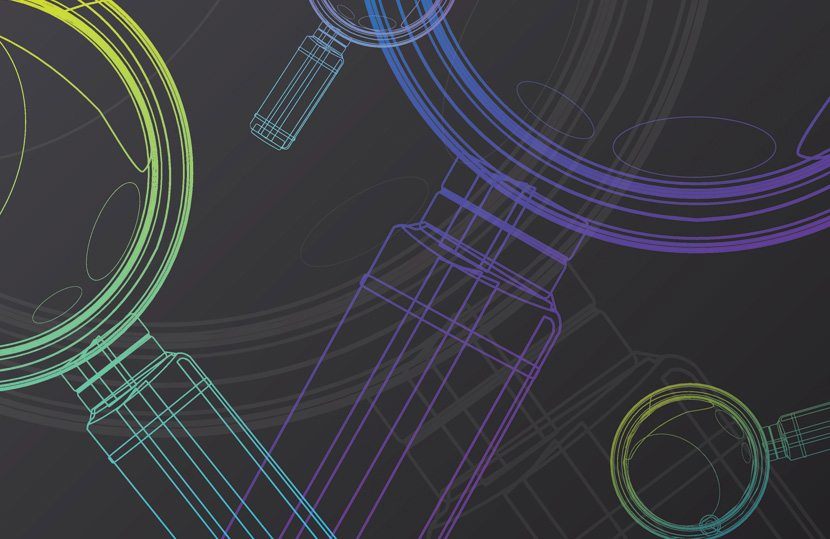
Image by: nidwlw, ©2017 Getty Images
Having a reliable and accurate enterprise search strategy within your organization boosts productivity internally and improves customer satisfaction externally. Both of these benefits come from providing fast, accurate, and relevant results at the time a search is performed. To fully understand how enterprise search works, its overall benefits, and what is required for a smooth performance, it is necessary to look at the key components and stages of an enterprise search strategy.
Define the Objectives and Scope for Search
An enterprise search, like a Google search, is designed to provide desirable and accurate results as quickly as possible. However, enterprise-based search varies drastically from its World Wide Web counterpart. For starters, business content often lacks links, text, or extensive context. In addition, there are various content formats to contend with beyond HTML. On top of that, all information searched and indexed within an enterprise network must go through several forms of security or authentication, limiting what some users can access. In addition, search results conducted within an enterprise network should offer the highest quality results, with the highest relevance first—not necessarily the most popular (though this may be a factor, particularly in a machine learning scenario). Because of these key differences, developing a search strategy must begin with defining its objectives."Users will not interact and feel engaged with a poorly thought-out solution."
Taxonomy
Creating a classification system for enterprise search, commonly referred to as taxonomy, is a complex topic. Companies can easily get hung up on how to approach it because their data is typically not categorized or naturally available in neat structures. The work to create these taxonomies requires time-consuming, manual intervention by a knowledgeable person in the organization. This work is necessary to create filters and facets to help the user.Recent advances in machine learning and artificial intelligence (AI) have provided hope for those looking to automate their taxonomy through auto-classification. Through machine learning, the system would understand the “data behind the data” over time, as the algorithm gets better at choosing which categories or tags belong with each piece of content.
User Experience (UX)
There are many features to an enterprise search solution, and the way it looks and how it is organized is critically important. Users will not interact and feel engaged with a poorly thought-out solution. Some of these features include providing an autosuggest with the keyword search box (using machine learning to determine what to suggest would improve the interaction as well); effect filters, facets, and sorting on the search results page so that a user could fine-tune what is being viewed to locate the most appropriate content; properly laid out links and text with descriptions and images to help the look and feel of the page; and, finally, the use of AI methods to personalize the search results.Tuning and Optimizing Enterprise Search
Creating an enterprise search strategy does not end with delivering the search results. The strategy needs to include how the organization is going to maintain and tune the results over time. The goal is to have the results improve over time rather than degrade. Without the correct resources and plans in place, it is very possible to get worse results as time goes on.To make sure this doesn’t happen, the right people and systems need to be put in place to test the system for both performance and accuracy. Technical and marketing staff should be working together continuously to improve the speed and effectiveness of the search. A lot of this information can be learned by thoroughly evaluating the search reports.
Search parameters can (and should) vary from one enterprise to the next, but the steps taken to create these parameters will remain the same. Regardless of industry or the amount of amassed data, utilizing these steps in creating and formulating an enterprise search strategy is important for internal productivity and customer satisfaction.
Christopher Risner is Co-Founder and Chief Strategy Officer of BlueBolt, Inc. Follow Chris on Twitter @cdrize.