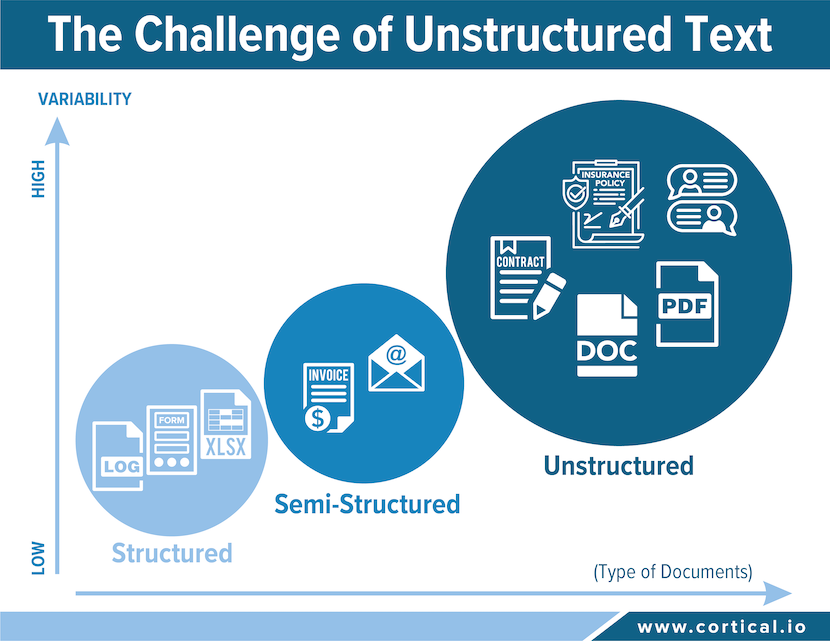
What is Intelligent Document Processing?
Many organizational processes are still dominated by paperwork. Even if most documents are now digitized, manual analysis of their content remains the rule. The high variability of content to review makes it impossible for robotic process automation tools to deliver reliable results.
Intelligent Document Processing (IDP) is a method of automating data extraction, analysis and processing that combines Artificial Intelligence (AI) techniques, like machine learning and Natural Language Processing (NLP), with tools like Optical Character Recognition (OCR) to keep human intervention to a minimum. For any company dealing with large quantities of information, finding an effective IDP solution is a great opportunity to improve process efficiencies, reduce costs and increase margins.
However, the market for IDP is largely dominated by solutions offering capabilities to process structured and semi-structured documents like invoices or customer onboarding forms. Processing more complex documents like insurance policies or content such as social media posts is still an emerging market because of the difficulty for legacy systems to understand the subtilities of human language.
This challenge can be solved with an innovative new NLP approach called Semantic Folding. Inspired by neuroscience, it powers a new breed of IDP solutions that efficiently and accurately process unstructured text, enabling new operational efficiencies in terms of time savings and increased responsiveness.
What Is Unstructured Data?
While the differences between structured, semi-structured, and structured text may seem complicated, it is critical to understand them when setting expectations and assessing IDP vendors.
The Limits of IDP Solutions The most common use cases for IDP solutions involve the processing of structured or semi-structured data like invoices, employee onboarding forms, or loan applications. Why IDP vendors focus on simple documents is obvious: this kind of organized, templated information is easy to feed into rule-based systems that are trained to extract pre-defined terms from pre-defined places.
Some IDP vendors address the challenge of semi-structured and unstructured text data by offering out-of-the-box solutions for very specific use cases, like contract processing or lease abstraction. They come mostly from the document capture industry and have leveraged the hundreds of thousands or even millions of documents in their database to train their systems. This has little to do with intelligent document processing, as these systems are not capable of processing documents that do not match a template in their repository.
Such IDP solutions deliver good results, provided the documents to process and company requirements exactly match the system parameters. However, whenever the documents used by a company differ from the templates, or the user needs go beyond simple term extractions, those systems fail to deliver accurate results.
Here are the two main reasons why IDP vendors struggle to offer solutions capable of generalizing the processing of unstructured text beyond very specific use cases:
1. The challenge of language variability
Corporate wording, product names, different ways of describing the same services, abbreviations, technical terminology, different vocabulary used by customers versus employees, etc.: the range of terms used within an organization is too broad to be captured as a whole, all the more as it evolves over time. The most advanced term-based NLP tools are not capable of processing terms they have not seen during training. Even if they have been trained with hundreds of billions of parameters, the training sets can’t possibly cover the complete spectrum of vocabulary used in a business context. In production, they perform poorly because they essentially ignore new terms. To improve their performance, these models would need to be trained with use-case specific data, which is all too often a scarce resource in the enterprise.
2. The challenge of language ambiguity
Human language is ambiguous, which means that the same concepts can be expressed in different ways. This is one of the main obstacles to automating the processing of unstructured text. Even advanced NLP algorithms are not capable of associating phrases with similar meaning but different wording. This is because they are based on word statistics, not high-level conceptual ideas.
How Semantic Folding Supercharges Your Unstructured Data
Semantic Folding leverages a new approach to natural language understanding by contextually understanding different meanings of words and recognizing various formulations of the same concept. For example, “we closed the deal” and “the contract is signed” are two different ways of formulating the same idea, but most IDP tools would fail at associating them. Not so with Semantic Folding-based IDP solutions which consequently deliver very high levels of accuracy in extracting key information and classifying content.
For example, group insurers need to review several complex documents like prior policies and competitors’ benefit booklets to prepare a quote for a company. The larger insurers employ between 50 to 100 employees to perform this job manually because they do not trust automation tools. A Semantic Folding solution can track important information that other tools would have missed because it accurately understands the meaning of certain formulations. For example, it recognizes that “Funding Method” and “Cost of Coverage” have the same meaning.
Accurately analyzing high volumes of emails with attachments is another typical challenge for IDP vendors where Semantic Folding approach makes a big difference. As an example, a large transportation company receiving hundreds of thousands of emails daily took advantage of the technology to filter out and flag urgent emails, and route them to the appropriate departments, thus dramatically improving response time and customer satisfaction.