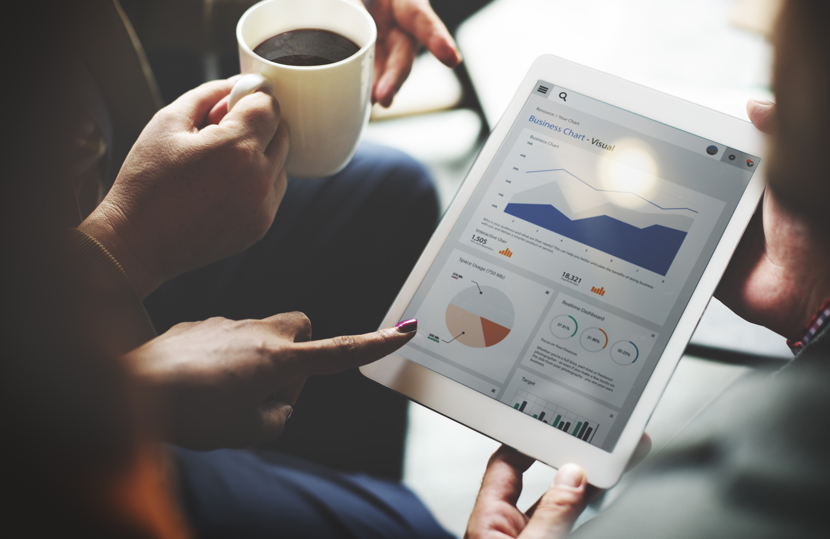
Image by: Rawpixel Ltd, ©2016 Getty Images
I had the honor of participating in a panel discussion about big data at the DOCUMENT Strategy Forum (DSF '16). The panel was moderated by Lane Severson from Doculabs, and I was joined by Carl Jaekel from Medical Mutual of Ohio and Declan Moss and Brett Collins from Navistar. We had a lively and very interactive discussion with lots of great audience participation. The only problem was we did not get very far on this very big topic, so I thought it would be useful to share some of my thoughts based on our discussion.
What is big data?
There are many definitions out there, but my definition of big data encompasses all content, which includes structured, semi-structured, and unstructured data. Before we get too far down the big data path, let’s make sure we have a set of definitions for these types of data. Structured data refers to information with a high degree of organization, such that inclusion in a relational database is seamless and readily searchable by simple, straightforward search engine algorithms or other search operations (like SQL). Examples include geospatial data, databases, and flat files. According to the Data Management Association (DAMA), unstructured data is any document, file, graphic, image, text, report, form, video, or sound recording that has not been tagged or otherwise structured into rows and columns or records, include with that emails and scanned paper (with optical character recognition and/or intelligent character recognition).
For me, the two biggest factors that get us to “big data” are volume and variety. The other thing to consider is not only the variety of data formats but also the data or information domain variety. For example, looking for relationships between financial data and geopolitical event data or looking across oil and gas market data, travel demand patterns, and airline routes for a specific geography become interesting challenges.
Can we really just turn big data on?
Yes, big data technology has developed and continues to improve regularly, but unfortunately, there is no magic wand here. We still have to know what questions to ask and ask them in a way that will generate meaningful and actionable answers. I think this is one of the biggest challenges of leveraging big data technologies and methodologies. There are three very important components to an effective big data program:
1. Effective Information Governance
For me, the two biggest factors that get us to “big data” are volume and variety. The other thing to consider is not only the variety of data formats but also the data or information domain variety. For example, looking for relationships between financial data and geopolitical event data or looking across oil and gas market data, travel demand patterns, and airline routes for a specific geography become interesting challenges.
Can we really just turn big data on?
Yes, big data technology has developed and continues to improve regularly, but unfortunately, there is no magic wand here. We still have to know what questions to ask and ask them in a way that will generate meaningful and actionable answers. I think this is one of the biggest challenges of leveraging big data technologies and methodologies. There are three very important components to an effective big data program:
1. Effective Information Governance
This includes identifying the systems of record and policies related to how data is handled and brought into the organization.
2. Established Data Owners
2. Established Data Owners
The only way the business is going to derive the value from their information is to be actively involved in managing it. If changes to the taxonomy are needed, who approves it? The business data owner should be the one that approves it. They are the ones who understand what the information is and the value to the organization.
3. Information Quality Processes
3. Information Quality Processes
To me, this is one of the most critical components to a successful big data effort. There needs to be well-defined procedures for how information enters the organization and how you communicate and train your people on these processes. This will prevent the “digital landfill" effect and will install the rigor required to make sure data quality remains high and that information delivers the value it should. Today’s big data tools and technologies cannot eliminate the effect of poor data quality. The adage of "garbage in, garbage out" still applies.
Ask the right questions
In my experience managing the information and data from the BP Gulf Oil Spill, knowing the right “questions” to ask about your data was one of the hardest areas as we looked to completely understand the vast amount of data and derive unknown insights from the data collected. You need business analysts who understand business intelligence and have analytics techniques and methods to take business stakeholders on a journey from an “outcome” back to the questions that would help achieve the outcome. From this, the queries and data needed to support those queries will allow for an associated answer to emerge.
Ask the right questions
In my experience managing the information and data from the BP Gulf Oil Spill, knowing the right “questions” to ask about your data was one of the hardest areas as we looked to completely understand the vast amount of data and derive unknown insights from the data collected. You need business analysts who understand business intelligence and have analytics techniques and methods to take business stakeholders on a journey from an “outcome” back to the questions that would help achieve the outcome. From this, the queries and data needed to support those queries will allow for an associated answer to emerge.
One thing big data technologies can do is help to discover and identify potential relationships and insights from large and varied amounts of data. The results of these initial discovery analytics can then be fed back to the business stakeholders to help them think about the questions that might be asked to achieve the desired outcomes via the answers we might get.
Where does unstructured data fit into the picture?
Much of the information with real business value is locked away inside documents, emails, and other unstructured data types. Full-text search alone may not generate the value the organization hoped for. In some cases, you may need to do some additional processing based on the questions and insights you are trying to answer and achieve.
An example of this is trying to extract the information value from invoices, which, in some cases, may be over 1,000 pages. Within that invoice, there may be 20 to 50 companies that have submitted invoices for payment to a prime contractor, who submitted it to the company presenting the invoice for payment. In many cases, big data technologies cannot fully understand this mess of unstructured data, which is even more unstructured. You get the picture.
This is a good example where preprocessing the data within these massive documents and turning it into structured data, which then can be better understood and analyzed using big data technologies, can deliver the desired results. This is not a one-size-fits-all scenario, so analysis must be carried out for each information set to see how far you can get using the current big data technologies.
For those readers who missed the big data panel at DSF '16, you missed a lively and great discussion. This is a big topic, and we could have gone on for several hours. My hope is that you have a good idea of some of my thoughts on big data and that our discussions at DSF '16 peaked your interest in delving further into this topic. One thing I can promise is that it will continue to evolve and the technologies will continue to get better and better. A trend to keep an eye on is how artificial intelligence will impact big data analytics.
Much of the information with real business value is locked away inside documents, emails, and other unstructured data types. Full-text search alone may not generate the value the organization hoped for. In some cases, you may need to do some additional processing based on the questions and insights you are trying to answer and achieve.
An example of this is trying to extract the information value from invoices, which, in some cases, may be over 1,000 pages. Within that invoice, there may be 20 to 50 companies that have submitted invoices for payment to a prime contractor, who submitted it to the company presenting the invoice for payment. In many cases, big data technologies cannot fully understand this mess of unstructured data, which is even more unstructured. You get the picture.
This is a good example where preprocessing the data within these massive documents and turning it into structured data, which then can be better understood and analyzed using big data technologies, can deliver the desired results. This is not a one-size-fits-all scenario, so analysis must be carried out for each information set to see how far you can get using the current big data technologies.
For those readers who missed the big data panel at DSF '16, you missed a lively and great discussion. This is a big topic, and we could have gone on for several hours. My hope is that you have a good idea of some of my thoughts on big data and that our discussions at DSF '16 peaked your interest in delving further into this topic. One thing I can promise is that it will continue to evolve and the technologies will continue to get better and better. A trend to keep an eye on is how artificial intelligence will impact big data analytics.
Russell Stalters is the founder of Clear Path Solutions Inc., author of gettinginformationdone.com and is a recognized information and data management expert. Previously, he was the director of information and data management and chief architect for BP’s Gulf Coast Restoration Organization. Follow him on Twitter @russellstalters.